The Role of Artificial Intelligence in Cloud Computing: A Comprehensive Guide
Artificial Intelligence (AI) transforms cloud computing, enabling smarter, more efficient, and scalable solutions for businesses and developers.
We’ll expand on the original content with technical details, real-world examples, and a critical analysis, providing a thorough resource for understanding this dynamic intersection in 2025.
What Is the Role of AI in Cloud Computing?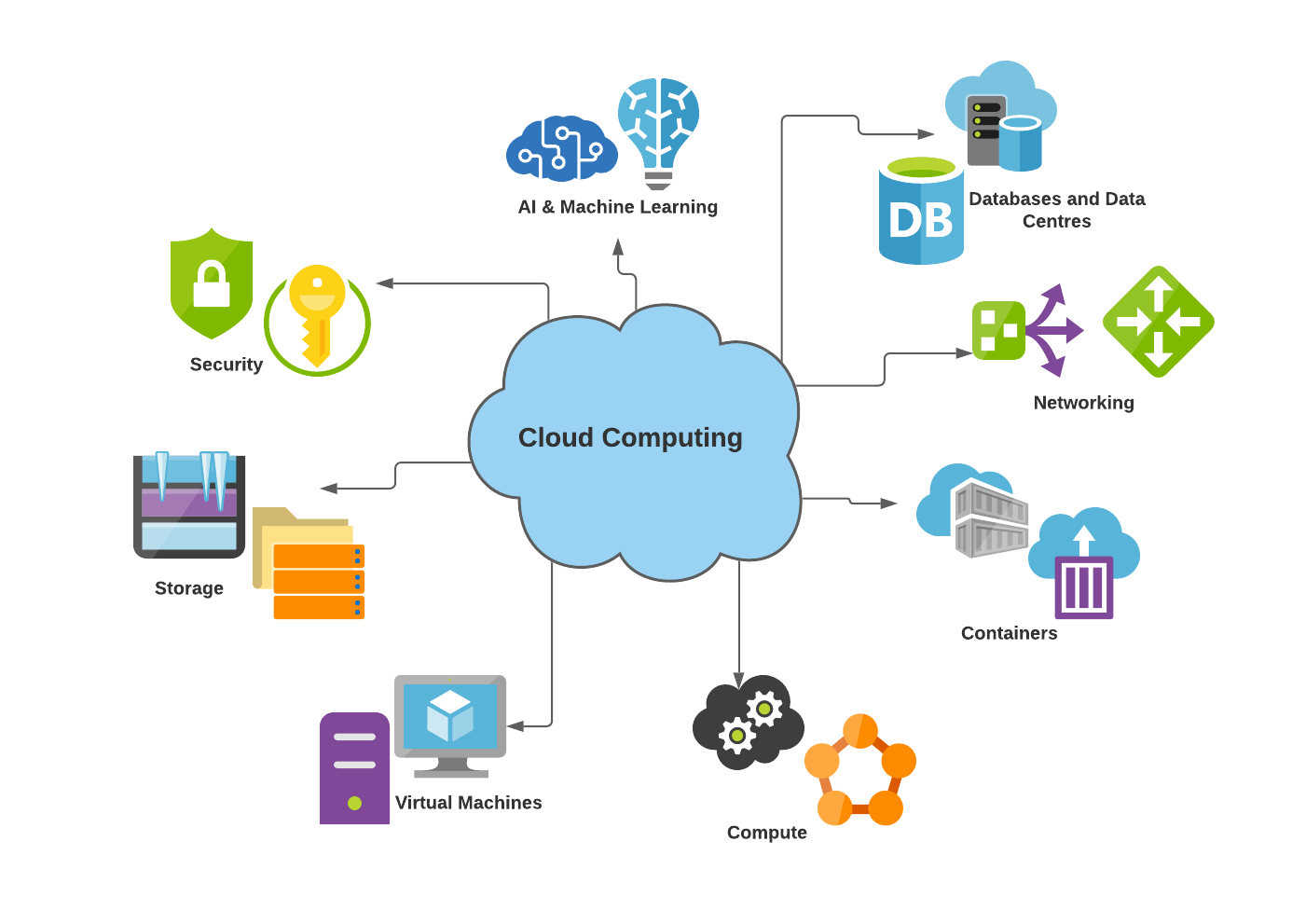
Cloud computing provides on-demand access to computing resources (e.g., servers, storage, databases) over the internet, while AI leverages algorithms and models to process data, make predictions, and automate tasks. AI enhances cloud platforms by optimizing resource management, improving user experiences, and enabling advanced analytics.
Key Roles of AI in Cloud Computing:
Resource Optimization: AI automates resource allocation, reducing waste and costs.
Data Analytics: AI processes massive datasets in the cloud for actionable insights.
Automation: AI streamlines operations like scaling, maintenance, and security.
Personalization: AI tailors cloud-based services to user preferences.
Security: AI detects and mitigates cyber threats in real time.
Example: Amazon Web Services (AWS) uses AI in its SageMaker platform to help developers build machine learning models, leveraging cloud scalability to process terabytes of data efficiently.
How AI Enhances Cloud Computing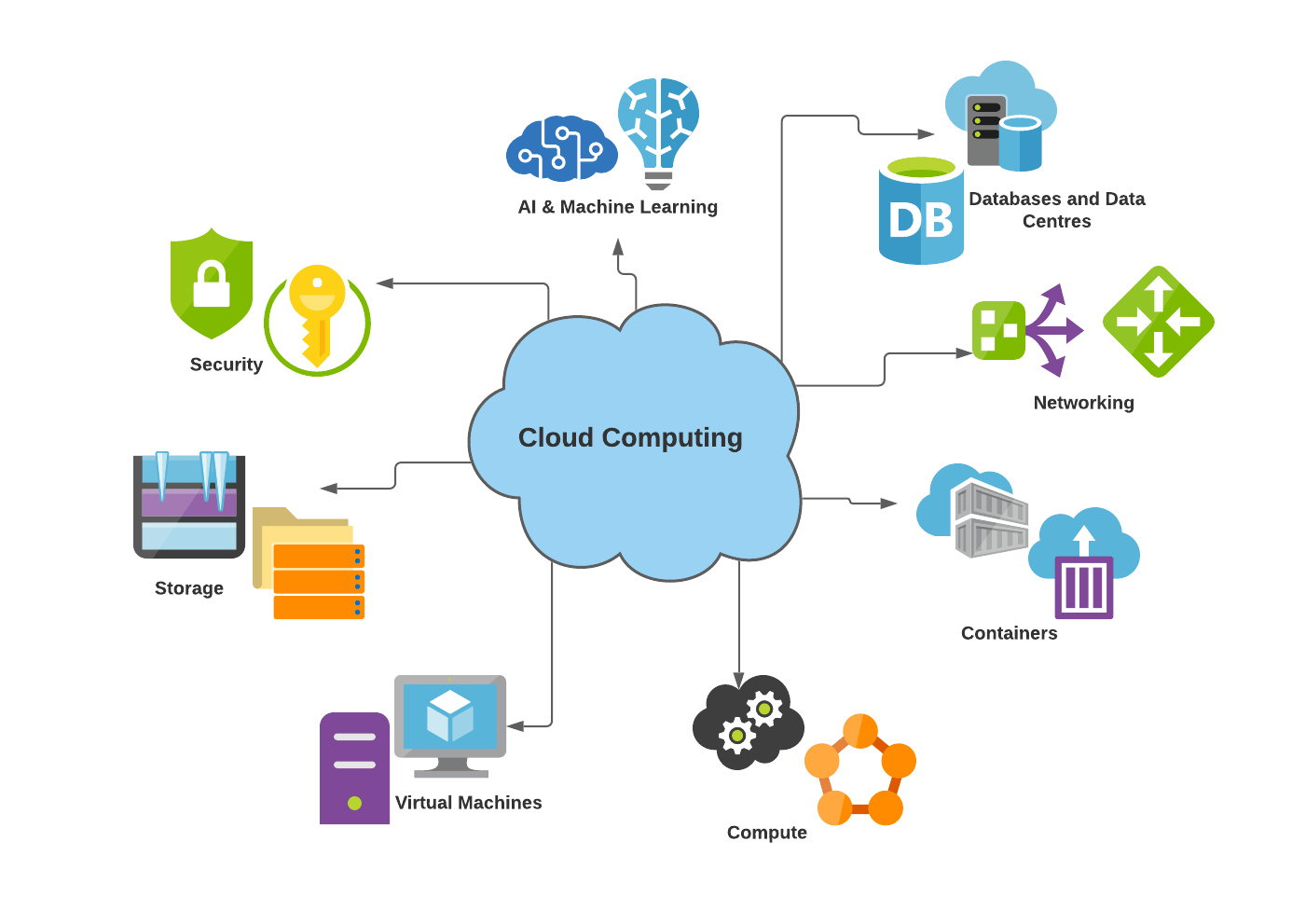
The original article highlights several ways AI contributes to cloud computing. Below, we expand on these points with technical insights, practical applications, and a critical analysis of their impact.
1. Optimized Resource Management
Explanation: AI algorithms dynamically predict demand and allocate cloud resources, ensuring efficiency and cost savings.
Expanded Details:
Predictive Scaling: AI models analyze historical usage patterns to forecast traffic spikes. For example, AWS Auto Scaling uses machine learning to adjust EC2 instances during peak e-commerce events like Black Friday.
Energy Efficiency: AI optimizes data center operations, reducing power consumption. Google Cloud’s DeepMind AI cut data center cooling costs by 40% by predicting temperature needs.
Use Case: A streaming service like Netflix uses AI-driven cloud scaling to handle millions of concurrent viewers, ensuring smooth playback without over-provisioning servers.
Analysis:
Pros: It reduces costs by minimizing idle resources, enhances performance during traffic surges, and supports sustainability by lowering energy use.
The cons are that it requires accurate training data, and poor predictions can lead to under- or over-scaling. The initial setup of AI models can be complex.
Relevance in 2025: With global data center energy consumption projected to reach 8% of electricity by 2030 (per IEA), AI-driven optimization is critical for cost and environmental goals.
Practical Tip: Use cloud-native AI tools (e.g., AWS Forecast, Azure Machine Learning) to model usage patterns. Monitor scaling logs to refine predictions over time.
2. Advanced Data Analytics
Explanation: AI processes vast amounts of cloud-stored data to uncover insights, driving business decisions and innovation.
Expanded Details:
Big Data Processing: AI frameworks like TensorFlow on Google Cloud analyze petabytes of data for patterns. For instance, retailers use AI to predict customer behavior based on purchase history stored in cloud data warehouses like Snowflake.
Real-Time Analytics: AI enables instant insights. Azure Stream Analytics with AI models processes IoT sensor data for predictive maintenance in manufacturing.
Use Case: A healthcare provider uses Google BigQuery with AI to analyze patient records, identifying trends in disease outbreaks within hours.
Analysis:
Pros: Accelerates decision-making with real-time insights. Scales effortlessly with cloud storage. Democratizes analytics for non-experts via AI tools.
The cons are high compute costs for large datasets and the requirement of robust governance for data privacy concerns (e.g., GDPR compliance).
Relevance in 2025: With global data creation expected to hit 181 zettabytes (per IDC), AI-powered cloud analytics is essential for industries like finance, healthcare, and retail.
Practical Tip: Use serverless analytics (e.g., AWS Athena) to reduce costs. Implement data anonymization to comply with privacy laws.
3. Automation of Cloud Operations
Explanation: AI automates repetitive tasks like backups, patching, and scaling, freeing developers for strategic work.
Expanded Details:
Infrastructure Management: AI tools like IBM Cloud Pak for Data automate database tuning and failover. AWS Systems Manager uses AI to schedule maintenance windows.
DevOps Integration: AI enhances CI/CD pipelines by predicting build failures. GitHub Copilot, hosted on Azure, suggests code optimizations in real time.
Use Case: A SaaS company uses AI-driven automation in AWS Lambda to deploy updates hourly, reducing manual intervention and downtime.
Analysis:
Pros: Saves time and reduces human error. Scales operations for large cloud environments. Lowers staffing costs for routine tasks.
Cons: Over-automation may reduce visibility into operations. AI misconfigurations can cause outages.
Relevance in 2025: As cloud adoption grows (80% of enterprises per Gartner 2024), automation is critical for managing complex, multi-cloud setups.
Practical Tip: Start with low-risk automation (e.g., backups) before automating critical tasks. Monitoring tools like Datadog can be used to track AI-driven operations.
4. Enhanced Security
Explanation: AI detects anomalies, predicts threats, and responds to cyberattacks in cloud environments faster than traditional methods.
Expanded Details:
Threat Detection: AI models analyze network traffic for suspicious patterns. Azure Sentinel uses AI to detect ransomware in real time.
Automated Response: AI triggers actions like isolating compromised instances. AWS GuardDuty quarantines threats automatically.
Use Case: A bank uses Google Cloud’s AI-powered Chronicle to monitor transactions, flagging fraudulent activity within seconds.
Analysis:
Pros: It improves response times to threats, scales security for large cloud deployments, and reduces reliance on manual monitoring.
Cons: False positives can disrupt legitimate operations. AI security tools require ongoing training to stay effective.
Relevance in 2025: With cyberattacks up 37% in 2024 (per X posts), AI-driven cloud security is a top business priority.
Practical Tip: Enable multi-factor authentication (MFA) alongside AI security tools. Regularly update threat models with new attack patterns.
5. Personalized User Experiences
Explanation: AI leverages cloud data to deliver tailored services, improving customer satisfaction and engagement.
Expanded Details:
Recommendation Systems: AI analyzes user behavior in cloud databases. AWS Personalize powers Amazon’s product recommendations, boosting sales.
Dynamic Content: AI customizes web content in real time. Firebase’s AI-driven Remote Config adjusts app interfaces based on user preferences.
Use Case: A music streaming app uses Azure AI to curate playlists, increasing user retention by 20%.
Analysis:
Pros: Enhances user engagement and revenue. Scales personalization for millions of users. Easy to integrate with cloud APIs.
Cons: Requires large datasets for accurate personalization. Privacy concerns arise from user data collection.
Relevance in 2025: With e-commerce and streaming dominating markets, personalized cloud services are a competitive edge.
Practical Tip: Use A/B testing to refine AI personalization models. Ensure transparent data usage policies to build user trust.
Challenges of AI in Cloud Computing
While AI enhances cloud computing, it introduces challenges:
Cost: AI workloads (e.g., training large models) can be expensive. Optimize with serverless or spot instances.
Complexity: Integrating AI requires expertise in cloud and ML frameworks. Invest in training or hire specialists.
Data Privacy: AI processing sensitive data raises compliance issues. Use encryption and anonymization.
Ethical Concerns: AI biases in analytics or personalization can harm users—audit models for fairness.
Dependency: Over-reliance on cloud AI tools risks vendor lock-in. Adopt open standards where possible.
Best Practices for Leveraging AI in Cloud Computing
To maximize AAI’s benefits in the cloud, follow these guidelines:
Start Small: Pilot AI with low-risk tasks (e.g., predictive scaling) before tackling complex analytics.
Optimize Costs: Use cost-effective instances (e.g., AWS Spot Instances) for AI training. Monitor spending with tools like Azure Cost Management.
Ensure Security: Encrypt data and use AI-driven threat detection. Comply with regulations like GDPR or CCPA.
Monitor Performance: Track AI model accuracy and usage of cloud resources with AWS CloudWatch or Google Stackdriver.
Mitigate Bias: Regularly audit AI models for fairness, especially in personalization or analytics.
Plan for Scalability: Design AI pipelines to handle growing data volumes, using serverless or distributed frameworks.
Conclusion
AI is revolutionizing cloud computing by optimizing resources, enabling advanced analytics, automating operations, enhancing security, and personalizing user experiences.
The original Shabakeh Magazine article provides a solid overview, but this guide enriches it with technical depth, real-world examples, and a critical analysis of benefits and challenges. In 2025, as data grows and businesses prioritize efficiency, AI-powered cloud solutions are indispensable for innovation and competitiveness.
From startups leveraging free-tier AI tools to enterprises securing global operations, AI and cloud computing synergy drive progress. By adopting best practices and addressing challenges, developers and businesses can unlock the full potential of this transformative combination.